AI-based discovery of methane mitigation additives for ruminants
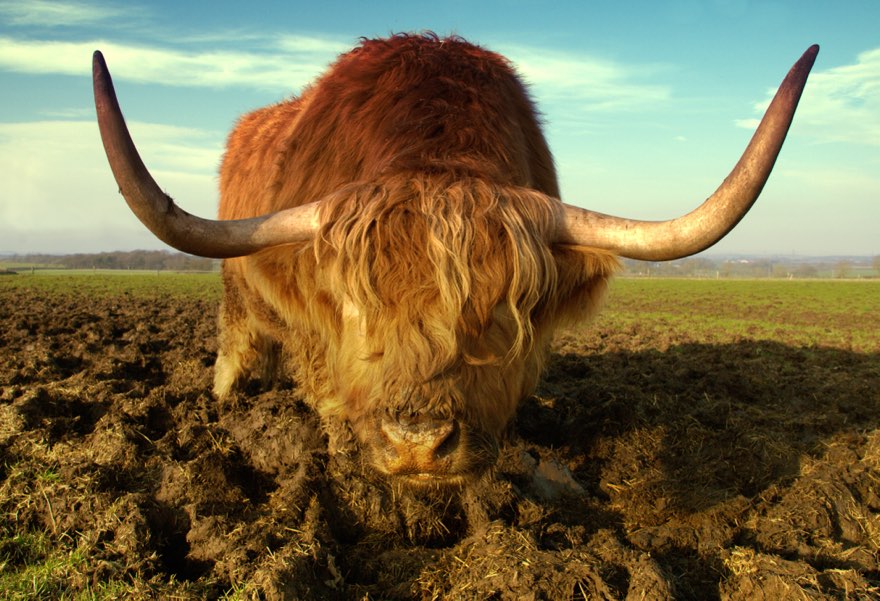
- Exclusive Offer for BSAS Members: 25% Discount on All CABI Books
- Minister Noel Grealish Officially Launches BSAS 2025 – The Leading Animal Science Conference Returns to Ireland
- Germinal Joins British Society of Animal Science as New Corporate Member
- Xinyi Huang Wins Prestigious Steve Bishop Award for Groundbreaking Bovine Mastitis De-tection Research
- View All
AI-based discovery of methane mitigation additives for ruminants
Lead supervisor: Professor Sokratis Stergiadis, School of Agriculture, Policy and Development, Department of Animal Sciences, University of Reading
Email: s.stergiadis@reading.ac.uk
Co-supervisors: Dr Katerina Theodoridou, School of Biological Sciences, Queen's University Belfast; Dr Mauricio Cafiero, Department of Chemistry, University of Reading
Project description: Methane emissions from ruminants, such as cattle and sheep, are a significant contributor to global greenhouse gas emissions, accounting for approximately 44% of human-made methane. These emissions not only impact the environment but also represent an energy loss in livestock production. This project tackles this urgent issue by developing innovative solutions to reduce methane emissions, contributing directly to global climate goals such as the Global Methane Pledge and the UK’s Net Zero Strategy.
The focus of the project is on discovering natural compounds that can inhibit a key enzyme, methyl-coenzyme M reductase (MCR), which is essential for methane production in the rumen. To achieve this, the research will employ advanced artificial intelligence (AI) tools to screen thousands of compounds for their potential to reduce methane production. These tools include molecular docking, machine learning, and molecular dynamics simulations, which will predict how well different compounds interact with the enzyme.
Promising compounds will undergo further testing in laboratory models that simulate the rumen environment, such as the Rumen Simulation Technique (RUSITEC), which mimics the fermentation processes within the rumen over an extended period. These tests will measure not only methane reduction but also impacts on fermentation efficiency and microbial communities, ensuring that selected compounds are effective and sustainable. The top-performing compounds will then be tested in animal trials with cattle, where methane emissions, feed efficiency, and animal health will be rigorously observed using state-of-the-art animal monitoring technologies.
This research represents a fusion of advanced AI techniques and applied agricultural science, offering a transformative approach to reducing agricultural emissions. The use of natural compounds ensures alignment with consumer and industry demands for sustainable and safe interventions. Furthermore, the outcomes of this project have the potential to deliver significant environmental benefits while maintaining or even enhancing livestock productivity. By advancing our understanding of methane mitigation and developing innovative feed additives, this project addresses one of the most pressing challenges in sustainable agriculture and offers a unique opportunity to contribute to impactful, solution-driven research with tangible benefits for the environment, industry, and society.
Training opportunities: The project offers multidisciplinary training, including technical and transferable skills essential for a successful research career. At the University of Reading, the student will gain expertise in AI-based molecular docking, quantum mechanical calculations, and machine learning models to identify methane inhibitors. Training will include the Rumen Simulation Technique for methane reduction analysis and gas chromatography methods for evaluating fermentation parameters. An 18-month placement at the Agri-Food and Biosciences Institute will provide hands-on experience in animal trials with beef cattle, including assessments of methane emissions, feed intake, growth and animal health. At Queen’s University Belfast, the student will develop skills in microbiome analysis and metagenomics to study shifts in rumen microbial populations during methane mitigation trials. Data analysis training will include linear mixed models and multivariate redundancy analysis. The student will also benefit from the University of Reading’s Graduate School workshops on research skills, scientific writing, and personal development.
Project supervision style: The student will be supported by a lead supervisor, two co-supervisors representing different disciplines, and an industry CASE partner supervisor. Weekly 1:1 meetings with the lead supervisor will monitor progress and address challenges. Monthly meetings with the full supervisory team, including the CASE partner, will ensure academic and industry alignment. During the student’s 18-month industry placement, bi-weekly virtual check-ins with the lead supervisor will maintain academic support, while weekly meetings with the industry supervisor will provide
guidance in the industrial setting. Additionally, the student will spend a few weeks training with the co-supervisor, receiving specialised guidance and participating in virtual meetings for ongoing support. Feedback on written work will be provided within two weeks, and for urgent deliverables, within one week. This structure ensures continuous communication, even during remote periods, and fosters interdisciplinary and applied learning.
Student profile: Applicants should hold at least an upper second-class degree in a relevant scientific discipline, such as animal science, biology, biochemistry, computational sciences, or related fields. A foundational understanding of animal metabolism, physiology, microbiology, organic chemistry or molecular structures is important for engaging with the project’s focus on methane mitigation in ruminants. Familiarity with computational tools (e.g., Python, R) and basic data analysis skills is beneficial, but not essential, as comprehensive training in AI-based molecular docking, machine learning
models, and advanced statistical methods will be provided. Similarly, prior experience with laboratory techniques, such as fermentation models (e.g., RUSITEC), gas chromatography, microbiome analysis, or animal trials, is advantageous but not required, as the student will receive hands-on training in these areas.
Candidates should demonstrate a willingness to develop both computational and biological research skills, as the project integrates innovative AI techniques with applied agricultural science.
Stipend (Salary): FoodBioSystems DTP students receive an annual tax free stipend (salary) that is paid in instalments throughout the year. For 2024/25 this is £19,237 (£21,237 at Brunel University) and it will increase slightly each year at rate set by UKRI.
Equity Diversity and Inclusion: The FoodBioSystems DTP is committed to equity, diversity and inclusion (EDI), to building a doctoral researcher(DR) and staff body that reflects the diversity of society, and to encourage applications from under-represented and disadvantaged groups. Our actions to promote diversity and inclusion are detailed on the FoodBioSystems DTP website and include:
• Offering reasonable adjustments at interview for shortlisted candidates who have disclosed a disability or specific learning difference.
• Guaranteed interview and applicant mentoring schemes for applicants, with UK home fees status, from eligible under-represented ethnic groups.